Neighborhood watch
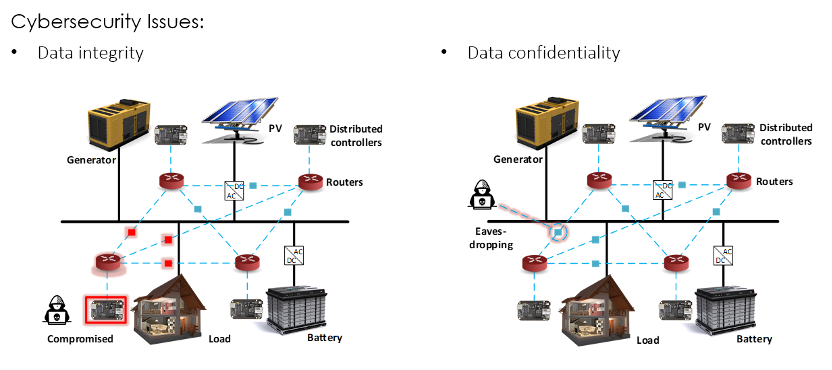
One of the pressing challenges in the distributed EMS field is cybersecurity. Energy system is one of the most critical infrastructures. Its security is of paramount significance to the well-being of the society. Most state-of-the-art distributed EMS algorithms lack security features that can withstand cyber threats, e.g. data integrity attacks. From our interaction with the microgrid operators, we learned that the cybersecurity of the system and the resilience against cyber-attacks are must-have features for the distributed DER controller. The two critical cybersecurity aspects of the distributed microgrid EMS are 1) data integrity; and 2) data confidentiality. Our lab has been investigating these cybersecurity issues since 2012.
Publications:
[1] Z. Cheng and M. -Y. Chow, “Resilient Collaborative Distributed Energy Management System Framework for Cyber-Physical DC Microgrids,” in IEEE Transactions on Smart Grid, vol. 11, no. 6, pp. 4637-4649, Nov. 2020, doi: 10.1109/TSG.2020.3001059.
[2] F. Ye, Z. Cheng, X. Cao and M. -Y. Chow, “A Random-Weighted Privacy-Preserving Distributed Algorithm for Energy Management in Microgrid with Energy Storage Devices,” 2020 2nd IEEE International Conference on Industrial Electronics for Sustainable Energy Systems (IESES), Cagliari, Italy, 2020, pp. 249-254, doi: 10.1109/IESES45645.2020.9210675.
[3] Z. Cheng and M. -Y. Chow, “An Augmented Bayesian Reputation Metric for Trustworthiness Evaluation in Consensus-based Distributed Microgrid Energy Management Systems with Energy Storage,” 2020 2nd IEEE International Conference on Industrial Electronics for Sustainable Energy Systems (IESES), Cagliari, Italy, 2020, pp. 215-220, doi: 10.1109/IESES45645.2020.9210638.
[4] Z. Cheng and M. Chow, “Reputation-based Collaborative Distributed Energy Management System Framework for Cyber-physical Microgrids: Resilience against Profit-driven Attacks,” 2020 IEEE Power & Energy Society Innovative Smart Grid Technologies Conference (ISGT), Washington, DC, USA, 2020, pp. 1-5, doi: 10.1109/ISGT45199.2020.9087737.
Sponsor:
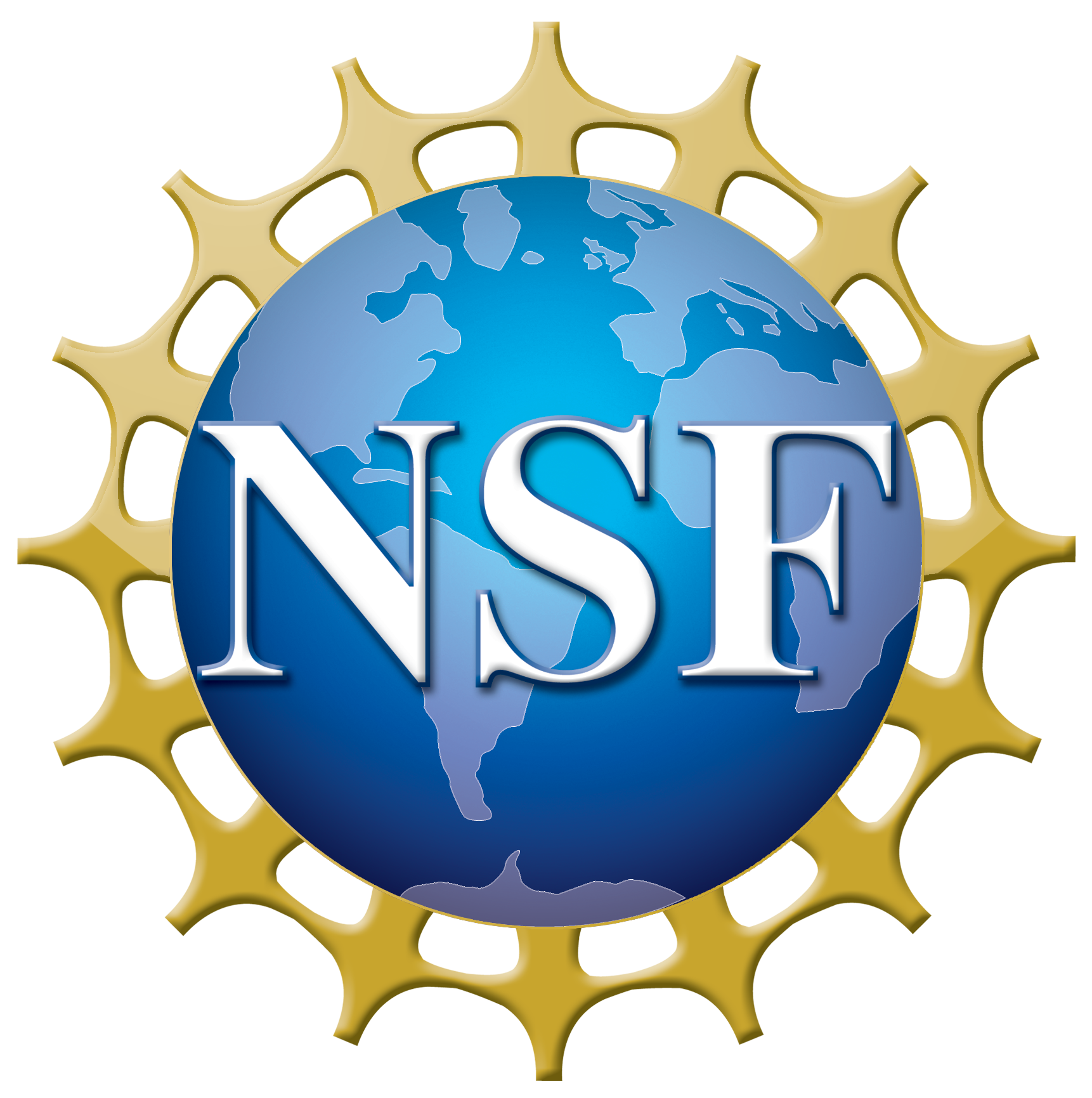