First Principle Based Four Dimensional Battery Degradation Model (4DM)
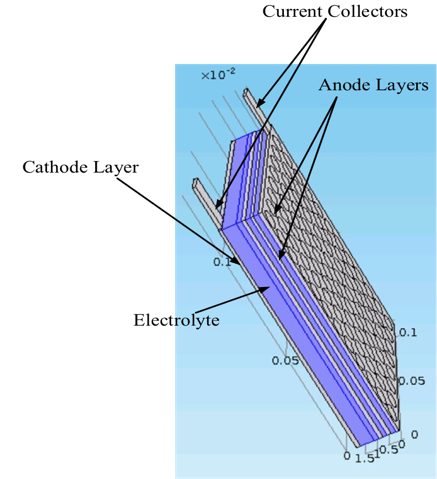
The First Principle Based Four Dimensional Battery Degradation Model (4DM) is computer simulation model for battery dynamics studies under different degradation and operating conditions. The 4DM is designed based on the physics of operation of the battery, i.e., the actual components such as anode, cathode, electrolyte, separator and current collector, are used to construct the model. This particular approach is used to bridge the gap between material science, electrochemical and electrical engineering.
The 4DM, because of the design, is capable of simulating:
- different battery chemistries,
- batteries of different capacities,
- progressive component degradation,
- different operating conditions – C-rates, temperatures, depth of discharge, partial charging and discharging effects,
- component degradation over time.
The 4DM provides a platform to study the sensitivity of the battery’s rate of change of voltage and capacity with respect to the degradation of different physical and electrochemical components. This feature/capability of the 4DM enables users to better understand the impact of different operating conditions on the degradation of their battery and determine appropriate use cases for their batteries to prolong the remaining useful life.
The 4DM has an intuitive user-interface that assists the user to perform different tests on the model under different operating conditions. The user interface is designed to be simple, yet intuitive and capable of providing the user with sufficient options to understand the working of the 4DM with access to the core back-end tool with all the features.
Sponsor: